Extra: Model training on partially paired data
In cases where we have paired multi-omics profiles for a subset of cells, such pairing information can also be utilized to improve integration accuracy. Below we will show how to instruct GLUE to utilize paired cells.
[1]:
from itertools import chain
from random import Random
import anndata as ad
import networkx as nx
import pandas as pd
import scanpy as sc
import scglue
import seaborn as sns
from matplotlib import rcParams
[2]:
scglue.plot.set_publication_params()
rcParams["figure.figsize"] = (4, 4)
Read preprocessed data
First, read the preprocessed data as produced by stage 1.
[3]:
rna = ad.read_h5ad("rna-pp.h5ad")
atac = ad.read_h5ad("atac-pp.h5ad")
guidance = nx.read_graphml("guidance.graphml.gz")
Label paired cells via obs_names
In scglue
, we use obs_names of the AnnData objects to identify paired cells across different modalities. Specifically, cells in different modalities with identical obs_name
s are treated as paired, while cells with different obs_name
s are treated as unpaired.
The dataset we are using is generated by SNARE-seq, which produces paired scRNA-seq and scATAC-seq profiles. In previous tutorials, we intentionally anonymized the paired cells by appending modality name to the cell barcodes (“_RNA”, “_ATAC”) to mimic an unpaired dataset:
[4]:
rna.obs_names, atac.obs_names
[4]:
(Index(['09L_GTTAGAACAGAT_RNA', '09I_CATAGCTCAACG_RNA', '09G_TTACAGGCAGGT_RNA',
'09B_CTATGGGCGTGC_RNA', '09L_AGTGATTGTTCG_RNA', '09C_ATATGTCTATAA_RNA',
'09A_CCTATTATTCGA_RNA', '09C_GCGTGCCGAATG_RNA', '09G_AGCTGACGCCGA_RNA',
'09D_CAGTCCCCAAAT_RNA',
...
'09H_GTTCGCTTGTCG_RNA', '09B_CAGGTGGTCTAT_RNA', '09C_GACCCTGTCGTT_RNA',
'09I_AACCAATCCTCA_RNA', '09C_AGAGTCTGAAAC_RNA', '09E_CCTGCTCGTCAC_RNA',
'09E_TGAGCACCTCCT_RNA', '09E_CCGGACTCGATC_RNA', '09F_GCCCGACTCGCA_RNA',
'09D_ATACTGCGCCCA_RNA'],
dtype='object', name='cells', length=9190),
Index(['09L_GTAAACCCGATA_ATAC', '09D_GCCGGGCGGCCG_ATAC',
'09J_TAGGCCTGAATT_ATAC', '09C_TCCGGCATCTCA_ATAC',
'09C_AGGCCACGTACA_ATAC', '09E_AAGGGGAGCTGG_ATAC',
'09K_CCGCCTTATAAT_ATAC', '09A_CGGATGTATCAT_ATAC',
'09B_TCAGAACTGCTA_ATAC', '09J_TCCGTGATAGAG_ATAC',
...
'09E_TAGTCGCGTACT_ATAC', '09J_GCACCTAAATGA_ATAC',
'09E_CACCCACGCTCC_ATAC', '09G_AACCATACGTTC_ATAC',
'09H_TGGCACAAAGGA_ATAC', '09H_GTTTACCTAGTT_ATAC',
'09F_TAGTTGGCAGAT_ATAC', '09L_CTTAGACGTCTA_ATAC',
'09C_GATGTAATTTGA_ATAC', '09I_TAGGCTTATTGT_ATAC'],
dtype='object', name='cells', length=9190))
Here we will de-anonymize 3000 cells to mimic a partially paired dataset.
Do NOT run the following code block when analyzing your own data. Instead, you just need to ensure that paired cells in your data have identical
obs_name
s, and unpaired cells have differentobs_name
s.
[5]:
rna_deanonym = rna.obs_names.str[:-4]
atac_deanonym = atac.obs_names.str[:-5]
assert set(rna_deanonym) == set(atac_deanonym) # The original barcodes are fully paired
paired_cells = set(Random(0).sample(set(rna_deanonym), 3000))
rna.obs_names = [
deanonym if deanonym in paired_cells else anonym
for anonym, deanonym in zip(rna.obs_names, rna_deanonym)
]
atac.obs_names = [
deanonym if deanonym in paired_cells else anonym
for anonym, deanonym in zip(atac.obs_names, atac_deanonym)
]
rna.obs_names, atac.obs_names
[5]:
(Index(['09L_GTTAGAACAGAT', '09I_CATAGCTCAACG_RNA', '09G_TTACAGGCAGGT',
'09B_CTATGGGCGTGC', '09L_AGTGATTGTTCG_RNA', '09C_ATATGTCTATAA_RNA',
'09A_CCTATTATTCGA_RNA', '09C_GCGTGCCGAATG_RNA', '09G_AGCTGACGCCGA',
'09D_CAGTCCCCAAAT_RNA',
...
'09H_GTTCGCTTGTCG_RNA', '09B_CAGGTGGTCTAT_RNA', '09C_GACCCTGTCGTT_RNA',
'09I_AACCAATCCTCA_RNA', '09C_AGAGTCTGAAAC_RNA', '09E_CCTGCTCGTCAC_RNA',
'09E_TGAGCACCTCCT_RNA', '09E_CCGGACTCGATC', '09F_GCCCGACTCGCA_RNA',
'09D_ATACTGCGCCCA_RNA'],
dtype='object', length=9190),
Index(['09L_GTAAACCCGATA_ATAC', '09D_GCCGGGCGGCCG_ATAC', '09J_TAGGCCTGAATT',
'09C_TCCGGCATCTCA_ATAC', '09C_AGGCCACGTACA', '09E_AAGGGGAGCTGG_ATAC',
'09K_CCGCCTTATAAT_ATAC', '09A_CGGATGTATCAT_ATAC', '09B_TCAGAACTGCTA',
'09J_TCCGTGATAGAG_ATAC',
...
'09E_TAGTCGCGTACT_ATAC', '09J_GCACCTAAATGA', '09E_CACCCACGCTCC',
'09G_AACCATACGTTC', '09H_TGGCACAAAGGA_ATAC', '09H_GTTTACCTAGTT',
'09F_TAGTTGGCAGAT_ATAC', '09L_CTTAGACGTCTA', '09C_GATGTAATTTGA_ATAC',
'09I_TAGGCTTATTGT'],
dtype='object', length=9190))
Configure data
When configuring the datasets, you need to set use_obs_names=True
in addition to other settings in stage 2. It tells the model to use obs_names
to identify paired cells:
[6]:
scglue.models.configure_dataset(
rna, "NB", use_highly_variable=True,
use_layer="counts", use_rep="X_pca",
use_obs_names=True
)
[7]:
scglue.models.configure_dataset(
atac, "NB", use_highly_variable=True,
use_rep="X_lsi",
use_obs_names=True
)
[8]:
guidance_hvf = guidance.subgraph(chain(
rna.var.query("highly_variable").index,
atac.var.query("highly_variable").index
)).copy()
Train GLUE model
We also use the scglue.models.fit_SCGLUE function to train GLUE model (see stage 2). The only difference is to set the model
argument to scglue.models.PairedSCGLUEModel
:
[9]:
glue = scglue.models.fit_SCGLUE(
{"rna": rna, "atac": atac}, guidance_hvf,
model=scglue.models.PairedSCGLUEModel,
fit_kws={"directory": "glue"}
)
[INFO] fit_SCGLUE: Pretraining SCGLUE model...
[INFO] autodevice: Using GPU 1 as computation device.
[INFO] check_graph: Checking variable coverage...
[INFO] check_graph: Checking edge attributes...
[INFO] check_graph: Checking self-loops...
[INFO] check_graph: Checking graph symmetry...
[INFO] PairedSCGLUEModel: Setting `graph_batch_size` = 27025
[INFO] PairedSCGLUEModel: Setting `max_epochs` = 222
[INFO] PairedSCGLUEModel: Setting `patience` = 19
[INFO] PairedSCGLUEModel: Setting `reduce_lr_patience` = 10
[INFO] PairedSCGLUETrainer: Using training directory: "glue/pretrain"
[INFO] PairedSCGLUETrainer: [Epoch 10] train={'g_nll': 0.448, 'g_kl': 0.005, 'g_elbo': 0.452, 'x_rna_nll': 0.166, 'x_rna_kl': 0.007, 'x_rna_elbo': 0.173, 'x_atac_nll': 0.04, 'x_atac_kl': 0.001, 'x_atac_elbo': 0.041, 'dsc_loss': 0.692, 'vae_loss': 0.243, 'gen_loss': 0.209, 'joint_cross_loss': 0.206, 'real_cross_loss': 0.208, 'cos_loss': 0.131}, val={'g_nll': 0.445, 'g_kl': 0.005, 'g_elbo': 0.45, 'x_rna_nll': 0.168, 'x_rna_kl': 0.008, 'x_rna_elbo': 0.176, 'x_atac_nll': 0.041, 'x_atac_kl': 0.001, 'x_atac_elbo': 0.042, 'dsc_loss': 0.693, 'vae_loss': 0.247, 'gen_loss': 0.212, 'joint_cross_loss': 0.208, 'real_cross_loss': 0.209, 'cos_loss': 0.142}, 6.3s elapsed
[INFO] PairedSCGLUETrainer: [Epoch 20] train={'g_nll': 0.431, 'g_kl': 0.004, 'g_elbo': 0.434, 'x_rna_nll': 0.163, 'x_rna_kl': 0.007, 'x_rna_elbo': 0.17, 'x_atac_nll': 0.04, 'x_atac_kl': 0.001, 'x_atac_elbo': 0.04, 'dsc_loss': 0.693, 'vae_loss': 0.238, 'gen_loss': 0.203, 'joint_cross_loss': 0.202, 'real_cross_loss': 0.205, 'cos_loss': 0.131}, val={'g_nll': 0.43, 'g_kl': 0.004, 'g_elbo': 0.434, 'x_rna_nll': 0.165, 'x_rna_kl': 0.007, 'x_rna_elbo': 0.172, 'x_atac_nll': 0.041, 'x_atac_kl': 0.001, 'x_atac_elbo': 0.041, 'dsc_loss': 0.694, 'vae_loss': 0.241, 'gen_loss': 0.206, 'joint_cross_loss': 0.205, 'real_cross_loss': 0.205, 'cos_loss': 0.144}, 6.8s elapsed
[INFO] PairedSCGLUETrainer: [Epoch 30] train={'g_nll': 0.424, 'g_kl': 0.004, 'g_elbo': 0.427, 'x_rna_nll': 0.162, 'x_rna_kl': 0.007, 'x_rna_elbo': 0.168, 'x_atac_nll': 0.039, 'x_atac_kl': 0.001, 'x_atac_elbo': 0.04, 'dsc_loss': 0.693, 'vae_loss': 0.236, 'gen_loss': 0.202, 'joint_cross_loss': 0.201, 'real_cross_loss': 0.204, 'cos_loss': 0.131}, val={'g_nll': 0.424, 'g_kl': 0.004, 'g_elbo': 0.427, 'x_rna_nll': 0.162, 'x_rna_kl': 0.007, 'x_rna_elbo': 0.169, 'x_atac_nll': 0.04, 'x_atac_kl': 0.001, 'x_atac_elbo': 0.04, 'dsc_loss': 0.694, 'vae_loss': 0.237, 'gen_loss': 0.202, 'joint_cross_loss': 0.204, 'real_cross_loss': 0.206, 'cos_loss': 0.147}, 6.6s elapsed
[INFO] PairedSCGLUETrainer: [Epoch 40] train={'g_nll': 0.42, 'g_kl': 0.004, 'g_elbo': 0.424, 'x_rna_nll': 0.16, 'x_rna_kl': 0.006, 'x_rna_elbo': 0.167, 'x_atac_nll': 0.039, 'x_atac_kl': 0.001, 'x_atac_elbo': 0.04, 'dsc_loss': 0.693, 'vae_loss': 0.234, 'gen_loss': 0.2, 'joint_cross_loss': 0.201, 'real_cross_loss': 0.204, 'cos_loss': 0.131}, val={'g_nll': 0.419, 'g_kl': 0.004, 'g_elbo': 0.423, 'x_rna_nll': 0.166, 'x_rna_kl': 0.006, 'x_rna_elbo': 0.172, 'x_atac_nll': 0.04, 'x_atac_kl': 0.001, 'x_atac_elbo': 0.04, 'dsc_loss': 0.694, 'vae_loss': 0.24, 'gen_loss': 0.206, 'joint_cross_loss': 0.204, 'real_cross_loss': 0.205, 'cos_loss': 0.153}, 6.7s elapsed
Epoch 00041: reducing learning rate of group 0 to 2.0000e-04.
Epoch 00041: reducing learning rate of group 0 to 2.0000e-04.
[INFO] LRScheduler: Learning rate reduction: step 1
[INFO] PairedSCGLUETrainer: [Epoch 50] train={'g_nll': 0.418, 'g_kl': 0.004, 'g_elbo': 0.421, 'x_rna_nll': 0.16, 'x_rna_kl': 0.006, 'x_rna_elbo': 0.167, 'x_atac_nll': 0.039, 'x_atac_kl': 0.001, 'x_atac_elbo': 0.04, 'dsc_loss': 0.693, 'vae_loss': 0.234, 'gen_loss': 0.199, 'joint_cross_loss': 0.199, 'real_cross_loss': 0.203, 'cos_loss': 0.128}, val={'g_nll': 0.418, 'g_kl': 0.004, 'g_elbo': 0.422, 'x_rna_nll': 0.161, 'x_rna_kl': 0.006, 'x_rna_elbo': 0.168, 'x_atac_nll': 0.041, 'x_atac_kl': 0.001, 'x_atac_elbo': 0.042, 'dsc_loss': 0.693, 'vae_loss': 0.237, 'gen_loss': 0.203, 'joint_cross_loss': 0.202, 'real_cross_loss': 0.205, 'cos_loss': 0.147}, 6.2s elapsed
[INFO] PairedSCGLUETrainer: [Epoch 60] train={'g_nll': 0.418, 'g_kl': 0.004, 'g_elbo': 0.421, 'x_rna_nll': 0.161, 'x_rna_kl': 0.006, 'x_rna_elbo': 0.167, 'x_atac_nll': 0.039, 'x_atac_kl': 0.001, 'x_atac_elbo': 0.04, 'dsc_loss': 0.693, 'vae_loss': 0.234, 'gen_loss': 0.2, 'joint_cross_loss': 0.199, 'real_cross_loss': 0.202, 'cos_loss': 0.128}, val={'g_nll': 0.417, 'g_kl': 0.004, 'g_elbo': 0.421, 'x_rna_nll': 0.161, 'x_rna_kl': 0.006, 'x_rna_elbo': 0.167, 'x_atac_nll': 0.04, 'x_atac_kl': 0.001, 'x_atac_elbo': 0.041, 'dsc_loss': 0.693, 'vae_loss': 0.236, 'gen_loss': 0.201, 'joint_cross_loss': 0.202, 'real_cross_loss': 0.204, 'cos_loss': 0.15}, 6.9s elapsed
Epoch 00067: reducing learning rate of group 0 to 2.0000e-05.
Epoch 00067: reducing learning rate of group 0 to 2.0000e-05.
[INFO] LRScheduler: Learning rate reduction: step 2
[INFO] PairedSCGLUETrainer: [Epoch 70] train={'g_nll': 0.417, 'g_kl': 0.004, 'g_elbo': 0.421, 'x_rna_nll': 0.161, 'x_rna_kl': 0.006, 'x_rna_elbo': 0.167, 'x_atac_nll': 0.039, 'x_atac_kl': 0.001, 'x_atac_elbo': 0.04, 'dsc_loss': 0.693, 'vae_loss': 0.234, 'gen_loss': 0.2, 'joint_cross_loss': 0.199, 'real_cross_loss': 0.203, 'cos_loss': 0.127}, val={'g_nll': 0.418, 'g_kl': 0.004, 'g_elbo': 0.421, 'x_rna_nll': 0.161, 'x_rna_kl': 0.006, 'x_rna_elbo': 0.167, 'x_atac_nll': 0.041, 'x_atac_kl': 0.001, 'x_atac_elbo': 0.041, 'dsc_loss': 0.693, 'vae_loss': 0.236, 'gen_loss': 0.202, 'joint_cross_loss': 0.203, 'real_cross_loss': 0.205, 'cos_loss': 0.153}, 6.2s elapsed
2022-08-26 16:36:30,211 ignite.handlers.early_stopping.EarlyStopping INFO: EarlyStopping: Stop training
[INFO] EarlyStopping: Restoring checkpoint "69"...
[INFO] fit_SCGLUE: Estimating balancing weight...
[INFO] estimate_balancing_weight: Clustering cells...
[INFO] estimate_balancing_weight: Matching clusters...
[INFO] estimate_balancing_weight: Matching array shape = (16, 20)...
[INFO] estimate_balancing_weight: Estimating balancing weight...
[INFO] fit_SCGLUE: Fine-tuning SCGLUE model...
[INFO] check_graph: Checking variable coverage...
[INFO] check_graph: Checking edge attributes...
[INFO] check_graph: Checking self-loops...
[INFO] check_graph: Checking graph symmetry...
[INFO] PairedSCGLUEModel: Setting `graph_batch_size` = 27025
[INFO] PairedSCGLUEModel: Setting `align_burnin` = 37
[INFO] PairedSCGLUEModel: Setting `max_epochs` = 222
[INFO] PairedSCGLUEModel: Setting `patience` = 19
[INFO] PairedSCGLUEModel: Setting `reduce_lr_patience` = 10
[INFO] PairedSCGLUETrainer: Using training directory: "glue/fine-tune"
[INFO] PairedSCGLUETrainer: [Epoch 10] train={'g_nll': 0.416, 'g_kl': 0.004, 'g_elbo': 0.419, 'x_rna_nll': 0.161, 'x_rna_kl': 0.006, 'x_rna_elbo': 0.167, 'x_atac_nll': 0.039, 'x_atac_kl': 0.001, 'x_atac_elbo': 0.04, 'dsc_loss': 0.688, 'vae_loss': 0.234, 'gen_loss': 0.2, 'joint_cross_loss': 0.2, 'real_cross_loss': 0.203, 'cos_loss': 0.133}, val={'g_nll': 0.414, 'g_kl': 0.004, 'g_elbo': 0.418, 'x_rna_nll': 0.161, 'x_rna_kl': 0.006, 'x_rna_elbo': 0.167, 'x_atac_nll': 0.04, 'x_atac_kl': 0.0, 'x_atac_elbo': 0.041, 'dsc_loss': 0.702, 'vae_loss': 0.235, 'gen_loss': 0.2, 'joint_cross_loss': 0.202, 'real_cross_loss': 0.206, 'cos_loss': 0.143}, 6.7s elapsed
[INFO] PairedSCGLUETrainer: [Epoch 20] train={'g_nll': 0.413, 'g_kl': 0.004, 'g_elbo': 0.417, 'x_rna_nll': 0.16, 'x_rna_kl': 0.006, 'x_rna_elbo': 0.166, 'x_atac_nll': 0.039, 'x_atac_kl': 0.0, 'x_atac_elbo': 0.04, 'dsc_loss': 0.69, 'vae_loss': 0.234, 'gen_loss': 0.199, 'joint_cross_loss': 0.2, 'real_cross_loss': 0.203, 'cos_loss': 0.134}, val={'g_nll': 0.414, 'g_kl': 0.004, 'g_elbo': 0.417, 'x_rna_nll': 0.163, 'x_rna_kl': 0.006, 'x_rna_elbo': 0.169, 'x_atac_nll': 0.041, 'x_atac_kl': 0.0, 'x_atac_elbo': 0.041, 'dsc_loss': 0.714, 'vae_loss': 0.238, 'gen_loss': 0.202, 'joint_cross_loss': 0.205, 'real_cross_loss': 0.206, 'cos_loss': 0.141}, 6.5s elapsed
[INFO] PairedSCGLUETrainer: [Epoch 30] train={'g_nll': 0.411, 'g_kl': 0.004, 'g_elbo': 0.415, 'x_rna_nll': 0.161, 'x_rna_kl': 0.006, 'x_rna_elbo': 0.167, 'x_atac_nll': 0.039, 'x_atac_kl': 0.0, 'x_atac_elbo': 0.04, 'dsc_loss': 0.689, 'vae_loss': 0.234, 'gen_loss': 0.199, 'joint_cross_loss': 0.2, 'real_cross_loss': 0.203, 'cos_loss': 0.135}, val={'g_nll': 0.412, 'g_kl': 0.004, 'g_elbo': 0.415, 'x_rna_nll': 0.163, 'x_rna_kl': 0.006, 'x_rna_elbo': 0.169, 'x_atac_nll': 0.041, 'x_atac_kl': 0.0, 'x_atac_elbo': 0.041, 'dsc_loss': 0.71, 'vae_loss': 0.238, 'gen_loss': 0.202, 'joint_cross_loss': 0.204, 'real_cross_loss': 0.206, 'cos_loss': 0.149}, 6.6s elapsed
[INFO] PairedSCGLUETrainer: [Epoch 40] train={'g_nll': 0.41, 'g_kl': 0.004, 'g_elbo': 0.413, 'x_rna_nll': 0.16, 'x_rna_kl': 0.006, 'x_rna_elbo': 0.166, 'x_atac_nll': 0.039, 'x_atac_kl': 0.0, 'x_atac_elbo': 0.04, 'dsc_loss': 0.694, 'vae_loss': 0.233, 'gen_loss': 0.198, 'joint_cross_loss': 0.2, 'real_cross_loss': 0.203, 'cos_loss': 0.135}, val={'g_nll': 0.41, 'g_kl': 0.004, 'g_elbo': 0.413, 'x_rna_nll': 0.164, 'x_rna_kl': 0.006, 'x_rna_elbo': 0.17, 'x_atac_nll': 0.04, 'x_atac_kl': 0.0, 'x_atac_elbo': 0.041, 'dsc_loss': 0.713, 'vae_loss': 0.239, 'gen_loss': 0.203, 'joint_cross_loss': 0.204, 'real_cross_loss': 0.204, 'cos_loss': 0.147}, 6.4s elapsed
[INFO] PairedSCGLUETrainer: [Epoch 50] train={'g_nll': 0.409, 'g_kl': 0.004, 'g_elbo': 0.412, 'x_rna_nll': 0.161, 'x_rna_kl': 0.006, 'x_rna_elbo': 0.166, 'x_atac_nll': 0.039, 'x_atac_kl': 0.0, 'x_atac_elbo': 0.04, 'dsc_loss': 0.689, 'vae_loss': 0.233, 'gen_loss': 0.199, 'joint_cross_loss': 0.199, 'real_cross_loss': 0.202, 'cos_loss': 0.136}, val={'g_nll': 0.409, 'g_kl': 0.004, 'g_elbo': 0.412, 'x_rna_nll': 0.164, 'x_rna_kl': 0.006, 'x_rna_elbo': 0.17, 'x_atac_nll': 0.041, 'x_atac_kl': 0.0, 'x_atac_elbo': 0.041, 'dsc_loss': 0.693, 'vae_loss': 0.239, 'gen_loss': 0.204, 'joint_cross_loss': 0.205, 'real_cross_loss': 0.207, 'cos_loss': 0.15}, 6.6s elapsed
[INFO] PairedSCGLUETrainer: [Epoch 60] train={'g_nll': 0.409, 'g_kl': 0.004, 'g_elbo': 0.412, 'x_rna_nll': 0.16, 'x_rna_kl': 0.006, 'x_rna_elbo': 0.166, 'x_atac_nll': 0.039, 'x_atac_kl': 0.0, 'x_atac_elbo': 0.04, 'dsc_loss': 0.692, 'vae_loss': 0.233, 'gen_loss': 0.198, 'joint_cross_loss': 0.199, 'real_cross_loss': 0.202, 'cos_loss': 0.135}, val={'g_nll': 0.409, 'g_kl': 0.004, 'g_elbo': 0.412, 'x_rna_nll': 0.163, 'x_rna_kl': 0.006, 'x_rna_elbo': 0.169, 'x_atac_nll': 0.04, 'x_atac_kl': 0.0, 'x_atac_elbo': 0.041, 'dsc_loss': 0.709, 'vae_loss': 0.238, 'gen_loss': 0.202, 'joint_cross_loss': 0.203, 'real_cross_loss': 0.206, 'cos_loss': 0.15}, 6.4s elapsed
[INFO] PairedSCGLUETrainer: [Epoch 70] train={'g_nll': 0.408, 'g_kl': 0.004, 'g_elbo': 0.412, 'x_rna_nll': 0.16, 'x_rna_kl': 0.006, 'x_rna_elbo': 0.165, 'x_atac_nll': 0.039, 'x_atac_kl': 0.0, 'x_atac_elbo': 0.04, 'dsc_loss': 0.687, 'vae_loss': 0.232, 'gen_loss': 0.198, 'joint_cross_loss': 0.199, 'real_cross_loss': 0.202, 'cos_loss': 0.136}, val={'g_nll': 0.409, 'g_kl': 0.003, 'g_elbo': 0.412, 'x_rna_nll': 0.16, 'x_rna_kl': 0.006, 'x_rna_elbo': 0.166, 'x_atac_nll': 0.04, 'x_atac_kl': 0.0, 'x_atac_elbo': 0.041, 'dsc_loss': 0.715, 'vae_loss': 0.234, 'gen_loss': 0.199, 'joint_cross_loss': 0.201, 'real_cross_loss': 0.203, 'cos_loss': 0.151}, 6.6s elapsed
[INFO] PairedSCGLUETrainer: [Epoch 80] train={'g_nll': 0.408, 'g_kl': 0.004, 'g_elbo': 0.412, 'x_rna_nll': 0.16, 'x_rna_kl': 0.006, 'x_rna_elbo': 0.166, 'x_atac_nll': 0.039, 'x_atac_kl': 0.0, 'x_atac_elbo': 0.04, 'dsc_loss': 0.692, 'vae_loss': 0.232, 'gen_loss': 0.198, 'joint_cross_loss': 0.199, 'real_cross_loss': 0.202, 'cos_loss': 0.135}, val={'g_nll': 0.408, 'g_kl': 0.004, 'g_elbo': 0.411, 'x_rna_nll': 0.164, 'x_rna_kl': 0.006, 'x_rna_elbo': 0.17, 'x_atac_nll': 0.04, 'x_atac_kl': 0.0, 'x_atac_elbo': 0.041, 'dsc_loss': 0.707, 'vae_loss': 0.238, 'gen_loss': 0.203, 'joint_cross_loss': 0.203, 'real_cross_loss': 0.206, 'cos_loss': 0.152}, 6.5s elapsed
Epoch 00081: reducing learning rate of group 0 to 2.0000e-04.
Epoch 00081: reducing learning rate of group 0 to 2.0000e-04.
[INFO] LRScheduler: Learning rate reduction: step 1
[INFO] PairedSCGLUETrainer: [Epoch 90] train={'g_nll': 0.407, 'g_kl': 0.003, 'g_elbo': 0.411, 'x_rna_nll': 0.16, 'x_rna_kl': 0.006, 'x_rna_elbo': 0.165, 'x_atac_nll': 0.039, 'x_atac_kl': 0.0, 'x_atac_elbo': 0.039, 'dsc_loss': 0.692, 'vae_loss': 0.232, 'gen_loss': 0.197, 'joint_cross_loss': 0.199, 'real_cross_loss': 0.201, 'cos_loss': 0.133}, val={'g_nll': 0.407, 'g_kl': 0.003, 'g_elbo': 0.41, 'x_rna_nll': 0.164, 'x_rna_kl': 0.006, 'x_rna_elbo': 0.17, 'x_atac_nll': 0.041, 'x_atac_kl': 0.0, 'x_atac_elbo': 0.041, 'dsc_loss': 0.708, 'vae_loss': 0.239, 'gen_loss': 0.203, 'joint_cross_loss': 0.206, 'real_cross_loss': 0.208, 'cos_loss': 0.148}, 6.7s elapsed
Epoch 00092: reducing learning rate of group 0 to 2.0000e-05.
Epoch 00092: reducing learning rate of group 0 to 2.0000e-05.
[INFO] LRScheduler: Learning rate reduction: step 2
[INFO] PairedSCGLUETrainer: [Epoch 100] train={'g_nll': 0.407, 'g_kl': 0.003, 'g_elbo': 0.41, 'x_rna_nll': 0.16, 'x_rna_kl': 0.006, 'x_rna_elbo': 0.166, 'x_atac_nll': 0.039, 'x_atac_kl': 0.0, 'x_atac_elbo': 0.04, 'dsc_loss': 0.689, 'vae_loss': 0.232, 'gen_loss': 0.198, 'joint_cross_loss': 0.199, 'real_cross_loss': 0.201, 'cos_loss': 0.133}, val={'g_nll': 0.407, 'g_kl': 0.003, 'g_elbo': 0.41, 'x_rna_nll': 0.161, 'x_rna_kl': 0.006, 'x_rna_elbo': 0.167, 'x_atac_nll': 0.04, 'x_atac_kl': 0.0, 'x_atac_elbo': 0.041, 'dsc_loss': 0.709, 'vae_loss': 0.235, 'gen_loss': 0.199, 'joint_cross_loss': 0.203, 'real_cross_loss': 0.207, 'cos_loss': 0.146}, 6.9s elapsed
Epoch 00103: reducing learning rate of group 0 to 2.0000e-06.
Epoch 00103: reducing learning rate of group 0 to 2.0000e-06.
[INFO] LRScheduler: Learning rate reduction: step 3
[INFO] PairedSCGLUETrainer: [Epoch 110] train={'g_nll': 0.407, 'g_kl': 0.003, 'g_elbo': 0.41, 'x_rna_nll': 0.16, 'x_rna_kl': 0.006, 'x_rna_elbo': 0.166, 'x_atac_nll': 0.039, 'x_atac_kl': 0.0, 'x_atac_elbo': 0.04, 'dsc_loss': 0.69, 'vae_loss': 0.232, 'gen_loss': 0.198, 'joint_cross_loss': 0.199, 'real_cross_loss': 0.201, 'cos_loss': 0.134}, val={'g_nll': 0.407, 'g_kl': 0.003, 'g_elbo': 0.41, 'x_rna_nll': 0.163, 'x_rna_kl': 0.006, 'x_rna_elbo': 0.168, 'x_atac_nll': 0.04, 'x_atac_kl': 0.0, 'x_atac_elbo': 0.04, 'dsc_loss': 0.712, 'vae_loss': 0.236, 'gen_loss': 0.201, 'joint_cross_loss': 0.203, 'real_cross_loss': 0.206, 'cos_loss': 0.151}, 8.4s elapsed
Epoch 00114: reducing learning rate of group 0 to 2.0000e-07.
Epoch 00114: reducing learning rate of group 0 to 2.0000e-07.
[INFO] LRScheduler: Learning rate reduction: step 4
[INFO] PairedSCGLUETrainer: [Epoch 120] train={'g_nll': 0.407, 'g_kl': 0.003, 'g_elbo': 0.41, 'x_rna_nll': 0.16, 'x_rna_kl': 0.006, 'x_rna_elbo': 0.165, 'x_atac_nll': 0.039, 'x_atac_kl': 0.0, 'x_atac_elbo': 0.04, 'dsc_loss': 0.689, 'vae_loss': 0.232, 'gen_loss': 0.198, 'joint_cross_loss': 0.199, 'real_cross_loss': 0.201, 'cos_loss': 0.133}, val={'g_nll': 0.407, 'g_kl': 0.003, 'g_elbo': 0.411, 'x_rna_nll': 0.162, 'x_rna_kl': 0.006, 'x_rna_elbo': 0.168, 'x_atac_nll': 0.04, 'x_atac_kl': 0.0, 'x_atac_elbo': 0.041, 'dsc_loss': 0.708, 'vae_loss': 0.236, 'gen_loss': 0.201, 'joint_cross_loss': 0.203, 'real_cross_loss': 0.205, 'cos_loss': 0.149}, 8.3s elapsed
Epoch 00128: reducing learning rate of group 0 to 2.0000e-08.
Epoch 00128: reducing learning rate of group 0 to 2.0000e-08.
[INFO] LRScheduler: Learning rate reduction: step 5
[INFO] PairedSCGLUETrainer: [Epoch 130] train={'g_nll': 0.407, 'g_kl': 0.003, 'g_elbo': 0.41, 'x_rna_nll': 0.16, 'x_rna_kl': 0.006, 'x_rna_elbo': 0.166, 'x_atac_nll': 0.039, 'x_atac_kl': 0.0, 'x_atac_elbo': 0.04, 'dsc_loss': 0.688, 'vae_loss': 0.232, 'gen_loss': 0.198, 'joint_cross_loss': 0.199, 'real_cross_loss': 0.201, 'cos_loss': 0.133}, val={'g_nll': 0.407, 'g_kl': 0.003, 'g_elbo': 0.411, 'x_rna_nll': 0.161, 'x_rna_kl': 0.006, 'x_rna_elbo': 0.167, 'x_atac_nll': 0.041, 'x_atac_kl': 0.0, 'x_atac_elbo': 0.042, 'dsc_loss': 0.716, 'vae_loss': 0.236, 'gen_loss': 0.2, 'joint_cross_loss': 0.202, 'real_cross_loss': 0.203, 'cos_loss': 0.146}, 7.0s elapsed
2022-08-26 16:54:00,388 ignite.handlers.early_stopping.EarlyStopping INFO: EarlyStopping: Stop training
[INFO] EarlyStopping: Restoring checkpoint "122"...
The resulting model will be an scglue.models.PairedSCGLUEModel object, but all functionalities remain the same.
Continue as in stage 2
From here on, you can continue following the instructions in stage 2 to obtain cell and feature embeddings:
[10]:
dx = scglue.models.integration_consistency(
glue, {"rna": rna, "atac": atac}, guidance_hvf
)
dx
[INFO] integration_consistency: Using layer "counts" for modality "rna"
[INFO] integration_consistency: Selecting aggregation "sum" for modality "rna"
[INFO] integration_consistency: Selecting aggregation "sum" for modality "atac"
[INFO] integration_consistency: Selecting log-norm preprocessing for modality "rna"
[INFO] integration_consistency: Selecting log-norm preprocessing for modality "atac"
[INFO] get_metacells: Clustering metacells...
[INFO] get_metacells: Aggregating metacells...
[INFO] metacell_corr: Computing correlation on 10 common metacells...
[INFO] get_metacells: Clustering metacells...
[INFO] get_metacells: Aggregating metacells...
[INFO] metacell_corr: Computing correlation on 20 common metacells...
[INFO] get_metacells: Clustering metacells...
[INFO] get_metacells: Aggregating metacells...
[INFO] metacell_corr: Computing correlation on 50 common metacells...
[INFO] get_metacells: Clustering metacells...
[INFO] get_metacells: Aggregating metacells...
[INFO] metacell_corr: Computing correlation on 100 common metacells...
[INFO] get_metacells: Clustering metacells...
[INFO] get_metacells: Aggregating metacells...
[INFO] metacell_corr: Computing correlation on 200 common metacells...
[10]:
n_meta | consistency | |
---|---|---|
0 | 10 | 0.173545 |
1 | 20 | 0.152228 |
2 | 50 | 0.112656 |
3 | 100 | 0.087442 |
4 | 200 | 0.067064 |
[11]:
_ = sns.lineplot(x="n_meta", y="consistency", data=dx).axhline(y=0.05, c="darkred", ls="--")
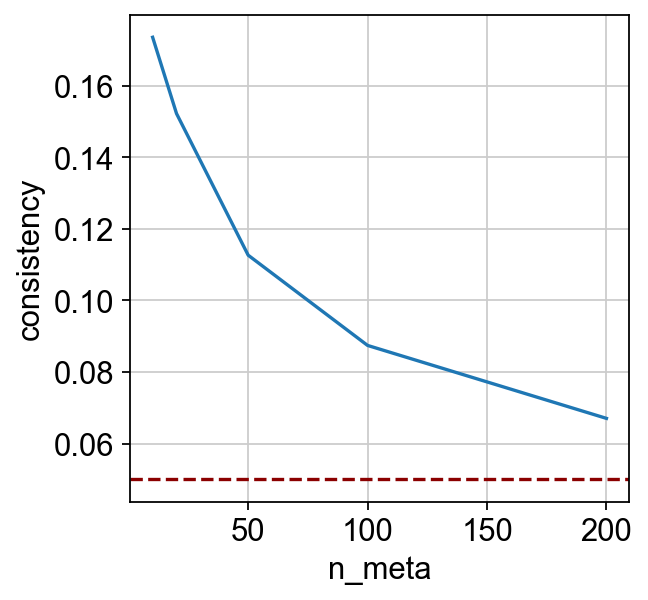
[12]:
rna.obsm["X_glue"] = glue.encode_data("rna", rna)
atac.obsm["X_glue"] = glue.encode_data("atac", atac)
[13]:
combined = ad.concat([rna, atac])
/rd2/user/caozj/GLUE/conda/lib/python3.8/site-packages/anndata/_core/anndata.py:1828: UserWarning: Observation names are not unique. To make them unique, call `.obs_names_make_unique`.
utils.warn_names_duplicates("obs")
[14]:
sc.pp.neighbors(combined, use_rep="X_glue", metric="cosine")
sc.tl.umap(combined)
sc.pl.umap(combined, color=["cell_type", "domain"], wspace=0.65)
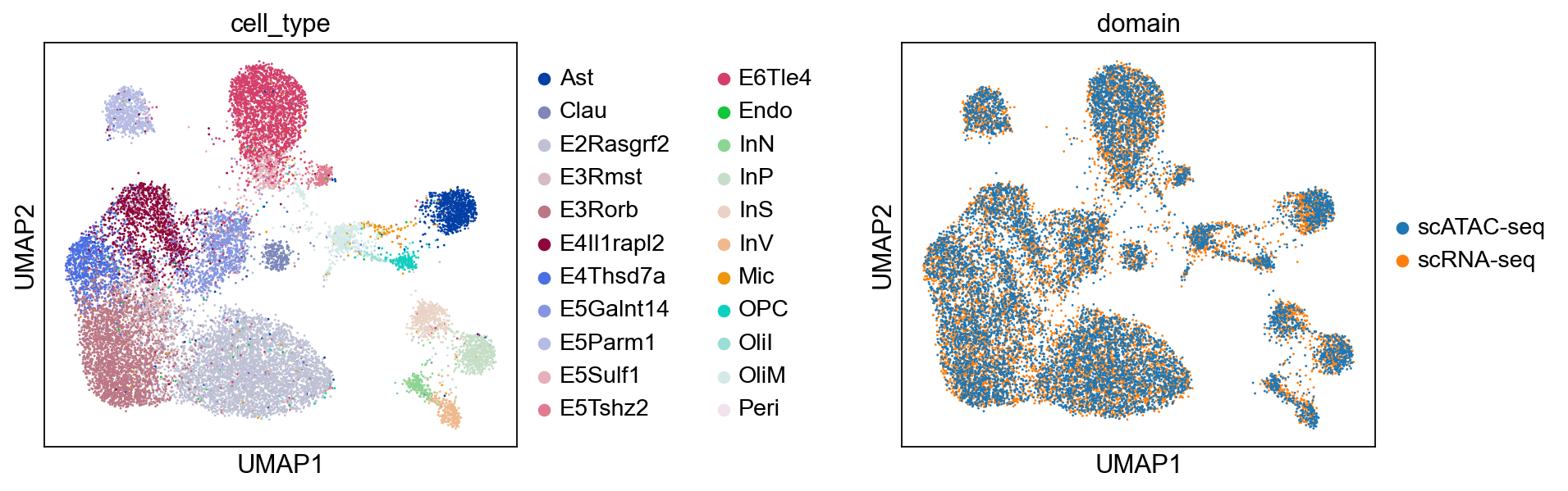
[15]:
feature_embeddings = glue.encode_graph(guidance_hvf)
feature_embeddings = pd.DataFrame(feature_embeddings, index=glue.vertices)
feature_embeddings.iloc[:5, :5]
[15]:
0 | 1 | 2 | 3 | 4 | |
---|---|---|---|---|---|
0610009B22Rik | -0.003289 | -0.101665 | 0.006089 | -0.005999 | -0.002966 |
0610025J13Rik | -0.002636 | 0.180847 | 0.000731 | 0.001941 | -0.006124 |
1110002J07Rik | -0.010751 | 0.079403 | 0.012048 | -0.011231 | -0.007835 |
1110006O24Rik | 0.003695 | -0.352514 | 0.000812 | -0.001779 | -0.003719 |
1110020A21Rik | -0.011558 | -0.251546 | 0.008463 | -0.003183 | -0.006828 |
[16]:
rna.varm["X_glue"] = feature_embeddings.reindex(rna.var_names).to_numpy()
atac.varm["X_glue"] = feature_embeddings.reindex(atac.var_names).to_numpy()